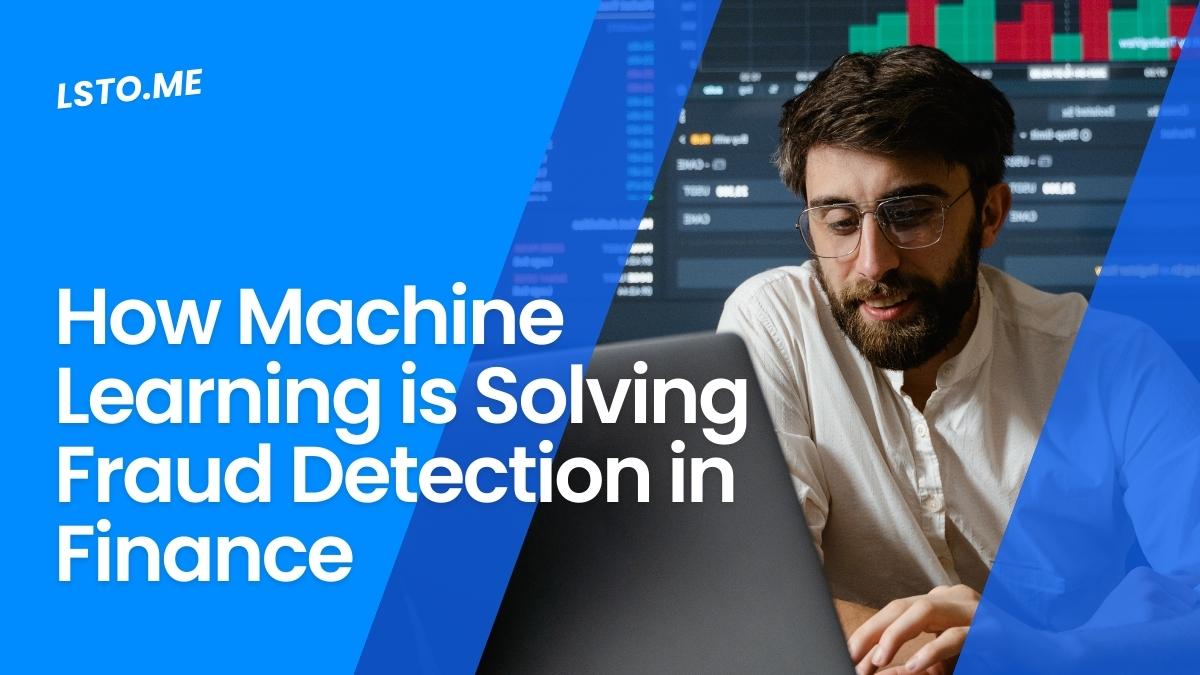
Fraud detection in finance has always been a challenging task due to the increasing complexity of fraud schemes and the volume of financial transactions taking place globally. However, with the advent of machine learning, fraud detection in finance has become more efficient and effective than ever before.
Machine learning is a subset of artificial intelligence that uses algorithms and statistical models to analyze and learn from data. By training on large datasets, machine learning algorithms can detect patterns and anomalies that are difficult or impossible for humans to identify. Here are some ways that machine learning is solving fraud detection in finance:
1. Real-time Fraud Detection
With the rise of digital transactions, fraud has become a significant problem for financial institutions. In response, machine learning algorithms have emerged as powerful tools for detecting and preventing fraudulent activities. Machine learning algorithms can analyze data in real-time to identify patterns that are indicative of fraud. By doing so, they can detect fraudulent activities as they occur and prevent them from causing further damage.
Machine learning algorithms work by analyzing large amounts of data to identify patterns that are not visible to the human eye. They use this information to create models that can accurately predict future events based on past behavior. This approach is particularly useful in finance because it allows institutions to quickly identify suspicious activity and take action before any damage is done. With real-time fraud detection, financial institutions can monitor transactions in real time, automatically flagging any unusual activity and alerting security teams if necessary.
2. Identification of Patterns and Anomalies
Machine learning algorithms have revolutionized the way we think about solving fraud detection in finance. Through sophisticated data analysis, machine learning models can identify patterns and anomalies that traditional methods may miss. By analyzing vast amounts of data, these algorithms can quickly detect any suspicious activity and alert authorities before significant damage occurs.
One of the key benefits of using machine learning in fraud detection is its ability to learn from past experiences. Machine learning algorithms can analyze huge volumes of historical data to understand what constitutes normal behavior for a particular financial transaction or account. This enables them to quickly detect any deviations from this norm and identify potential cases of fraud or financial irregularities.
Another important advantage of incorporating machine learning into fraud detection is its adaptability. As new forms of fraudulent activity emerge, machine learning models can quickly adapt and develop new rules to detect these anomalies in real time.
3. Reduction of False Positives
Fraud detection is a critical aspect of the financial industry. However, traditional fraud detection methods have been known to generate an overwhelming number of false positives. This can lead to a significant waste of time and resources as companies try to investigate each case thoroughly. In recent years, machine learning has emerged as a solution that can help reduce the number of false positives generated by traditional fraud detection methods.
Machine learning uses algorithms to analyze data and identify patterns that indicate fraudulent activity. By leveraging large volumes of data, machine learning systems can learn from past transactions and improve their accuracy over time. This means that they can quickly adapt to new fraud techniques and reduce the number of false positives generated by traditional fraud detection methods.
The adoption of machine learning in finance has led to increased efficiency in detecting fraudulent activities while reducing costs associated with investigating false alarms.
4. Predictive Analytics
Machine learning algorithms can be incredibly powerful tools for solving fraud detection problems in the finance industry. By analyzing historical data, these algorithms can identify patterns and anomalies that might indicate fraudulent activity. This is known as predictive analytics, and it’s a technique that is becoming increasingly popular in the world of finance.
One of the key advantages of using machine learning algorithms for fraud detection is their ability to handle large amounts of data. Traditional methods of fraud detection relied heavily on manual review processes, which can be time-consuming and prone to error. Machine learning algorithms can sift through vast quantities of data quickly and accurately, allowing them to identify potential fraud before it occurs.
Another advantage of using machine learning for fraud detection is its ability to adapt over time. As new types of fraudulent activity emerge, machine learning algorithms can learn from this information and adjust their approach accordingly.
5. Unsupervised Learning
Machine learning algorithms have revolutionized many industries, including finance. One area where machine learning is particularly useful is in solving fraud detection problems. Traditional fraud detection methods are often rule-based and rely on predefined patterns to identify fraud. However, with the increasing volume and complexity of financial transactions, these methods are no longer sufficient.
Unsupervised learning is a type of machine learning that can help overcome this challenge. Unlike supervised learning, which requires labeled data for training, unsupervised learning algorithms can identify new and previously unknown fraud patterns. This makes it a powerful tool for detecting increasingly sophisticated types of fraud.
With unsupervised learning, the algorithm can analyze large amounts of data without any prior knowledge or guidance about what to look for. It uses clustering techniques to group similar transactions and identify outliers that may be indicative of fraudulent activity.
Conclusion
Machine learning is revolutionizing fraud detection in finance by providing real-time detection, identifying patterns and anomalies, reducing false positives, enabling predictive analytics, and leveraging unsupervised learning. Financial institutions that adopt machine learning for fraud detection will be better equipped to prevent fraud and protect themselves and their customers from financial losses.